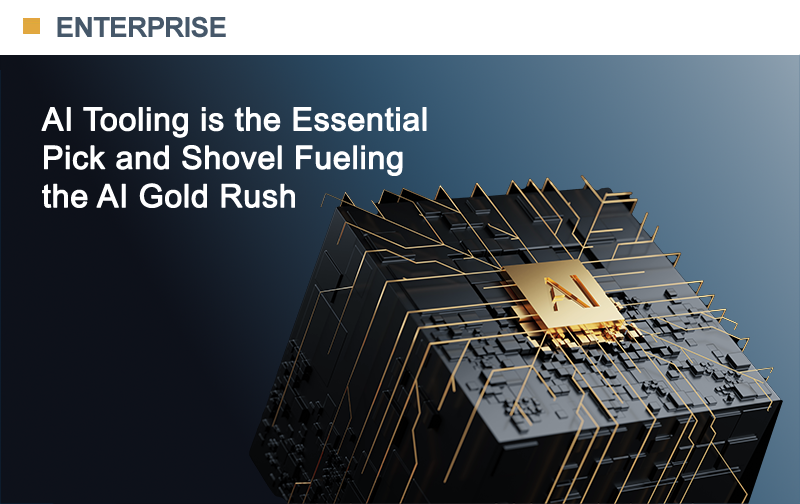
By Eric Lee, Principal, Cota Capital
The AI revolution is in full swing. Success stories of early AI adopters gaining competitive advantages have created a sense of urgency among business leaders. In fact, according to the 2024 State of the CIO survey, AI has replaced security as the top area of involvement for CIOs. This year, 80% of CIOs cite AI as their primary focus, up from 55% in 2023.
And these CIOs are increasingly putting their money where their mouth is. Worldwide spending on AI, including AI-enabled applications, infrastructure and related IT and business services, is projected to more than double by 2028 to $632 billion, according to IDC.
Sectors like financial services, healthcare, and manufacturing are leading the charge, but AI is making inroads across virtually every industry. Businesses are tapping into AI for everything from customer service chatbots and personalized marketing to predictive maintenance in manufacturing and fraud detection in finance. Meanwhile, the launch of OpenAI’s ChatGPT sparked an unprecedented surge of corporate interest in large language models (LLMs) and generative AI.
The AI tech stack and the potential in AI tooling
As AI continues to evolve, we can expect to see even more innovative use cases emerge, further fueling adoption and investment. At Cota Capital, we are particularly interested in AI infrastructure. Enterprises invested heavily in the modern AI stack this year, and this is expected to continue. According to an IDC survey, spending on AI infrastructure for generative AI workloads has skyrocketed, now outpacing both cybersecurity and the modernization of storage and data management as the top IT budget priority. The AI technology stack comprises several layers:
- Data Center / Hardware: Data centers and silicon-level components like Nvidia chips
- Cloud Platforms: Services like AWS, Google Cloud, and Microsoft Azure that provide access to computing power
- AI Models: Foundation models or LLMs from companies like OpenAI and Anthropic
- AI Tooling: The software, platforms, and frameworks that enable developers to create, fine-tune, and deploy AI models and apps
- AI Applications: End-user products built on these models
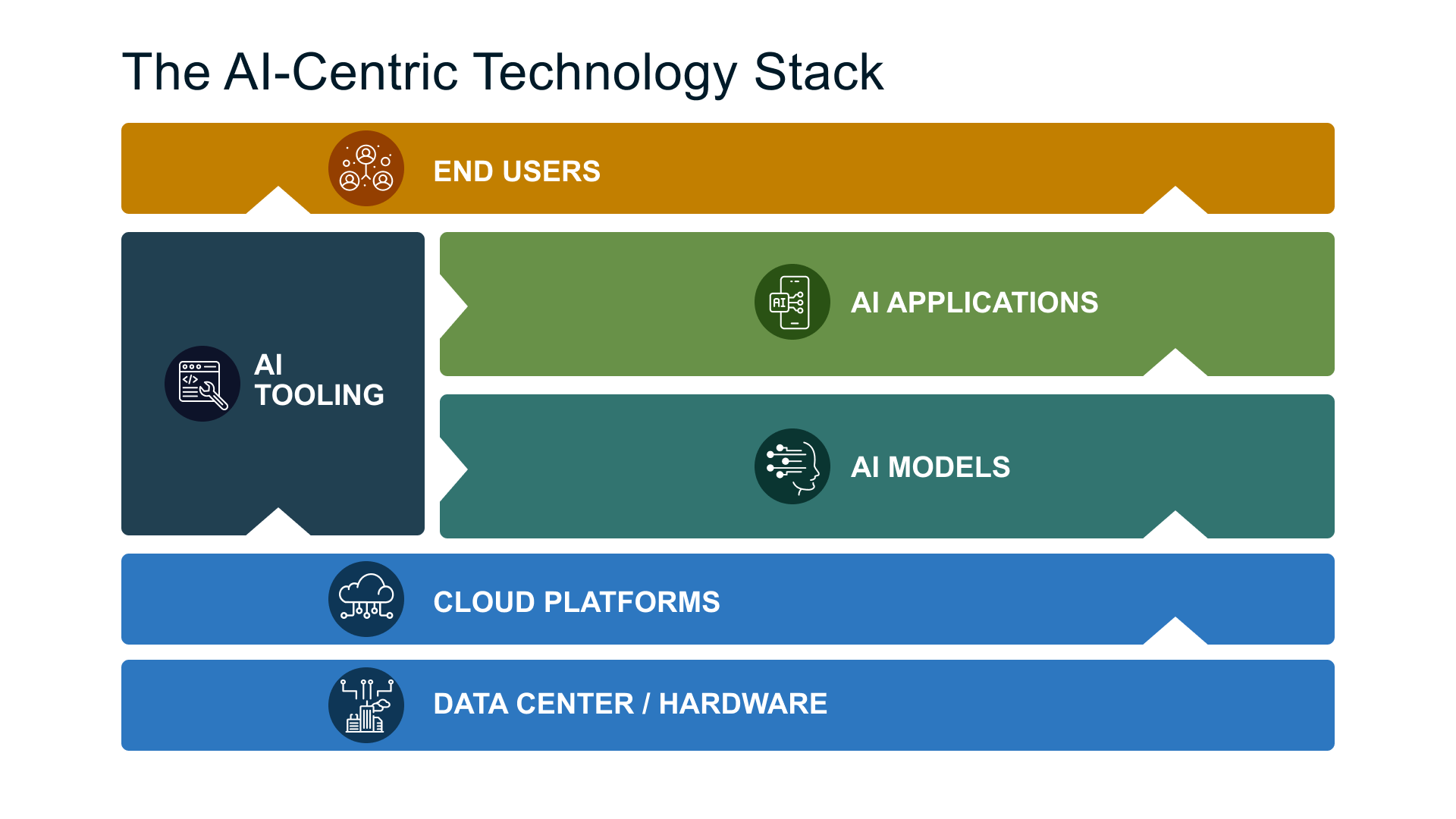
While large companies dominate the lower layers of this stack, there’s significant opportunity for startups in AI tooling in particular. These tools are crucial for supporting the entire AI lifecycle, from data preparation and model training to deployment and monitoring, enabling the creation of AI applications efficiently and effectively. As AI becomes more prevalent, the demand for specialized, user-friendly tools is likely to grow, creating space for innovative startups to thrive.
10 AI tooling subsectors
Let’s take a closer look at the 10 subsectors within AI tooling. We believe there are varying degrees of opportunity for startups in each of these subsectors.
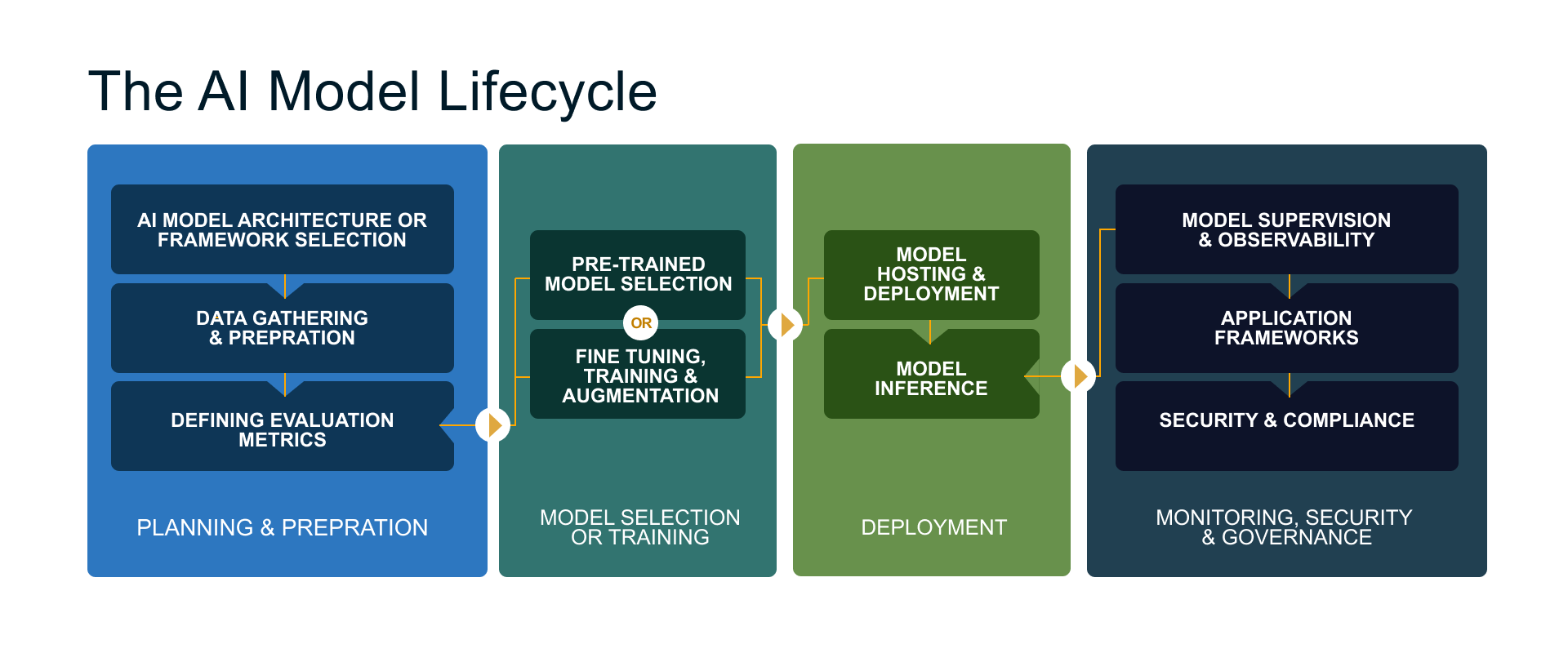
1: AI model architecture or framework selection: This is the crucial first step in building or training a model because it provides a solid foundation for moving forward. The framework chosen orchestrates the AI development lifecycle, including data collection, embedding, storage, model training, fine-tuning, logging, API integration, and validation.
2: Data gathering and preparation: Data is the key input for training these models and improving their performance. This stage requires scrubbing and cleansing the data to make it “model-ready,” ensuring it’s in an optimal state for use. This process requires various elements: data labeling, annotations, curation, loaders, storage, and retrieval systems. All of these components are crucial when trying to prepare the data needed to train and fine-tune an AI model for a specific use case.
3: Defining evaluation metrics: After all the data is prepared, a method must be defined to assess whether the AI model is functioning properly. This evaluation process is crucial for validating the model’s performance and effectiveness.
4: Pre-trained model selection: Some companies will choose to build their models from scratch. Others choose a pre-trained model, such as OpenAI, Anthropic, Mistral, or Llama, depending on the application’s requirements. Factors to consider include the size of the model, its performance on benchmark tasks, the resources required for fine-tuning, and the nature of the data on which it was originally trained.
5: Fine-tuning, training, and augmentation: Once a pre-trained model is selected, the next step in building an AI model is picking a fine-tuning approach. Fine-tuning involves adjusting the pre-trained model to perform a specific task. This subsector encompasses methods like low-rank adaptation, quantizing models, and few-shot learning, as well as retrieval augmented generation (RAG), which can enhance the model’s performance on specific tasks.
6: Model hosting & deployment: Once the model is prepared, the next step is to host it, which can be in a company’s private cloud or on a hyperscaler like AWS or Google Cloud. Some developers choose edge infrastructure operators like Cloudflare or deploying models directly on devices such as sensors, cameras, or IoT devices. Publishing the trained model to a cloud service allows it to be accessed and exploited by programs and put into production.
7: Model inference: Once the model is hosted, it enters the output layer where inference occurs. At this stage, all training has been completed, and the model is ready to be used for inferring or outputting specific information or processes. This is where the model actually produces results based on live data.
8: Model supervision & observability: This is where solutions come in to observe your model and ensure your AI stack is running properly. These tools are similar to application monitoring and troubleshooting solutions but specifically tailored for AI systems. They assess the model’s performance to ensure it functions as expected and makes accurate predictions.
9: Application frameworks: Next is building end-user applications leveraging the AI models and frameworks that have been set up. AI capabilities are linked to business apps and procedures at the application layer. Developers can create apps that use the predictions and suggestions made by the AI models and incorporate AI insights into the decision-making process.
10: Security & compliance: These tools are guardrails for protecting LLMs from external attacks or misuse by enforcing security policies, detecting and mitigating risks, and securing data and systems.
Fueling progress: our enthusiasm for AI tooling
We’re drawn to AI tooling startups for several key reasons. First off, AI tooling represents a net new opportunity in tech. There is no clear reference architecture when it comes to AI tooling. Unlike traditional software development, there’s no established “best practice” for building, training, and implementing generative AI applications. This leaves significant room for startups to define new standards and create novel products. We like the fact that there is a lack of established players, creating an open playing field. Many legacy incumbents weren’t built for this new AI-driven world, creating a vacuum that innovative startups can fill.
Additionally, as the “picks and shovels,” AI tooling companies benefit from the overall AI boom without being dependent on any one specific application’s success. Startups can become deeply entrenched in a company’s workflow, providing clear and tangible ROI for customers, which fosters vendor lock-in and increases switching costs.
Now that we’ve laid the groundwork and explored the key AI Tooling subsectors, stay tuned as our next post dives headfirst into the exciting world of AI Security!